Our paper On Outlier Exposure with Generative Models has been accepted on the NeurIPS Machine Learning Safety Workshop.
Abstract §
While Outlier Exposure reliably increases the performance of Out-of-Distribution detectors, it requires a set of available outliers during training. In this paper, we propose Generative Outlier Exposure (GOE), which alleviates the need for available outliers by using generative models to sample synthetic outliers from low-density regions of the data distribution. The approach requires no modification of the generator, works on image and text data, and can be used with pre-trained models. We demonstrate the effectiveness of generated outliers on several image and text datasets, including ImageNet.
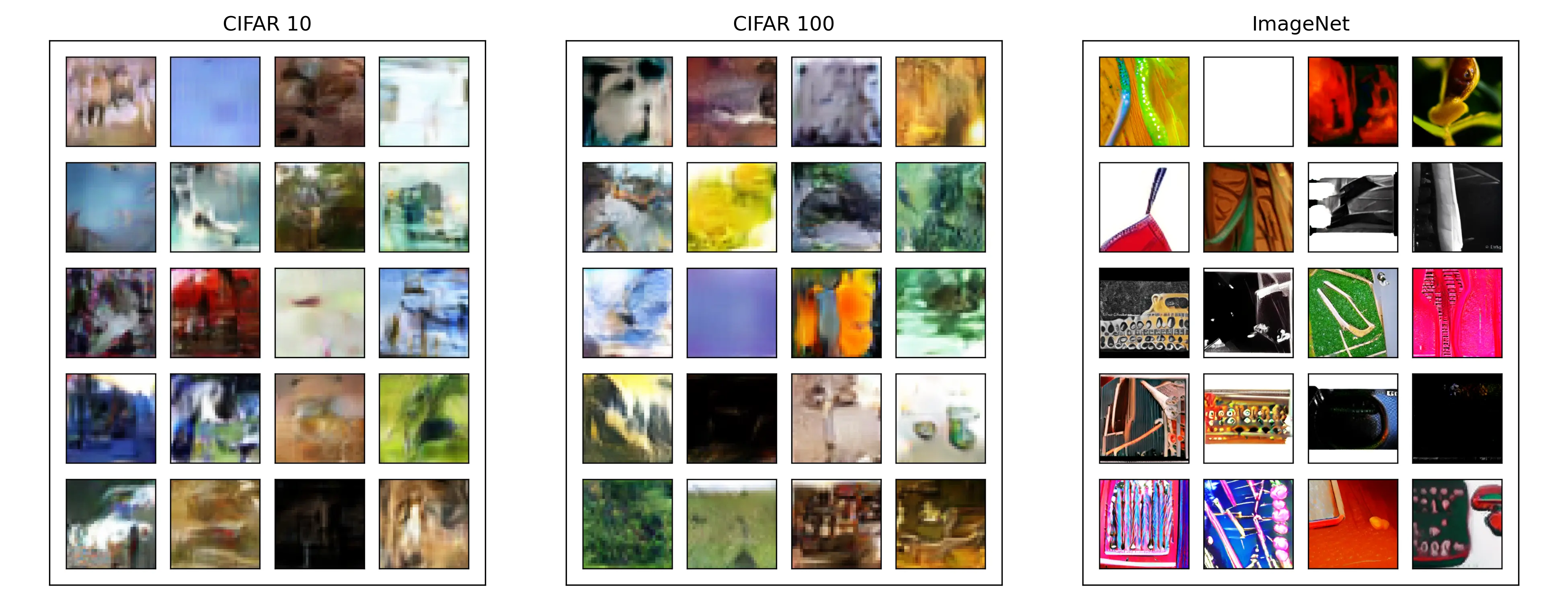
Outliers generated by BigGAN trained on different datasets