Our paper Deep learning-based harmonization and super-resolution of Landsat-8 and Sentinel-2 images, which is based on the masters thesis of my colleague Venkatesh Thirugnana Sambandham, has been published in the ISPRS Journal of Photogrammetry and Remote Sensing. This work is an extension of our previous workshop paper on transformers for satellite homogenization. In summary, we find that a simple UNet model provides surprisingly good performance for the satellite homogenization task.
We demonstrate that this 100M parameter model
- can enhance the spatial resolution of satellite images
- is able to increase the availability of cloud-free images by 21% on average
- can thereby provide benefits for downstream tasks, like crop segmentation
- generalizes well to different regions of the world
- is able to provide uncertainty estimates
The model is also available on Huggingface, so you can easily test it on your own images:
from transformers import AutoModel
model = AutoModel.from_pretrained(
"venkatesh-thiru/s2l8h-UNet-5depth-upsample",
trust_remote_code=True
)
Abstract §
Multi-spectral satellite images of the Earth’s surface are used in various applications, from water quality assessment and urban planning to climate monitoring, disaster response, infrastructure oversight, and agricultural surveillance. Many of these applications would benefit from higher spatial and temporal resolution of observations, which could be achieved by combining observations from several sources. This study introduces a deep learning-based pipeline to harmonize the spectral and spatial discrepancies between the Landsat-8 and Sentinel-2 Earth Observation satellites. Through established image quality metrics, we demonstrate a significant enhancement in the spatial resolution of Landsat-8 images. Field observation experiments show that leveraging unified images from both satellites increases the availability of cloud-free images by 21% annually on average in our study area. Additionally, our pipeline enhances the Normalized Difference Vegetation Index (NDVI) correlation between Landsat-8 and Sentinel-2 observations by about 4.9%, offering significant performance gains in a downstream crop segmentation task. Our 100M parameter model, trained on European data, generalizes to most regions with only minor limitations. Furthermore, we show that the pipeline can provide uncertainty estimates for its outputs, which are valuable for decision-making in downstream applications.
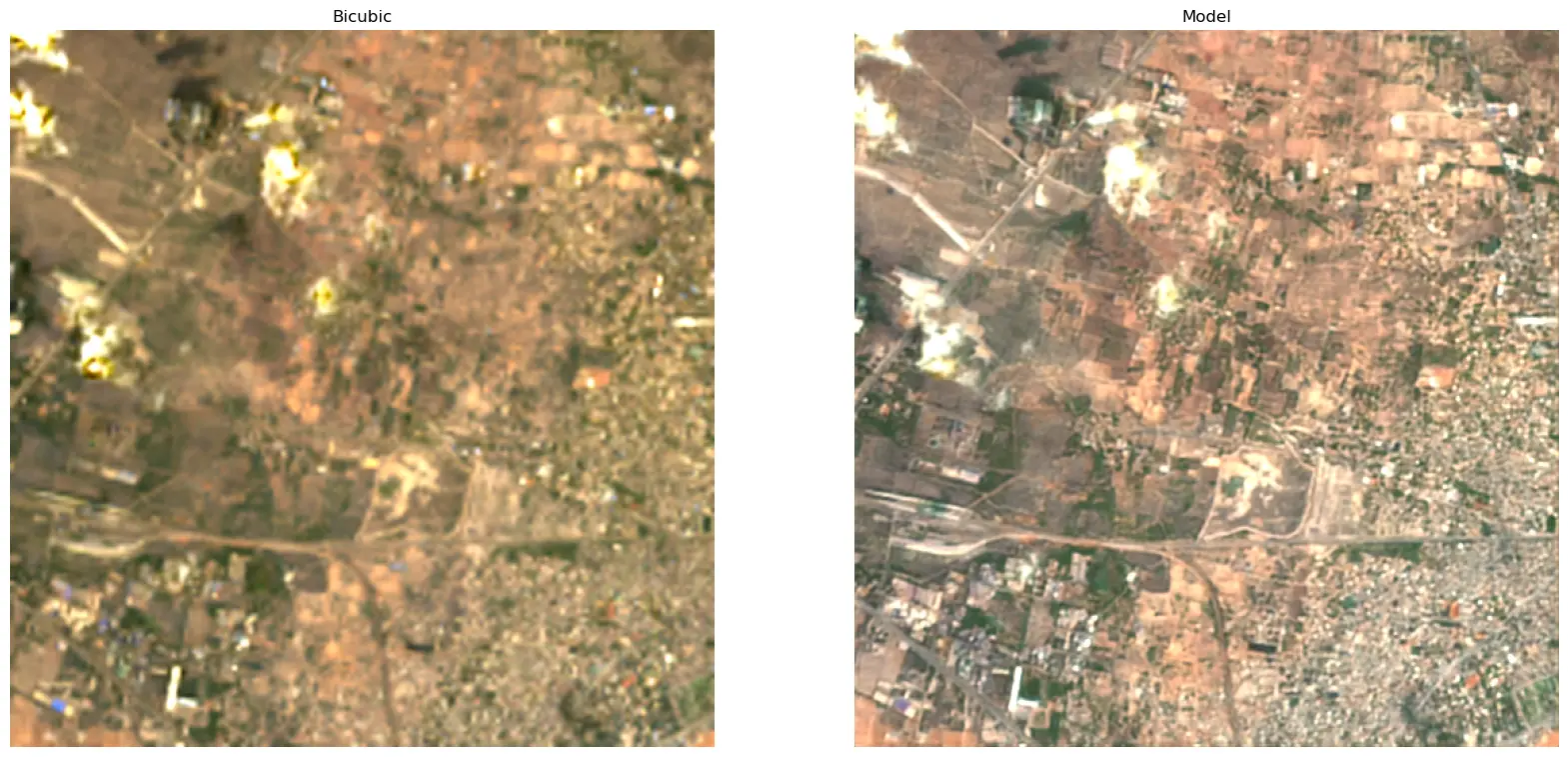
Satelite images upsampled by our model compared to baseline