Konstantin Kirchheim
I am currently a PhD student at the Otto-von-Guericke University Magdeburg (Germany) in the Department of Computer Science.
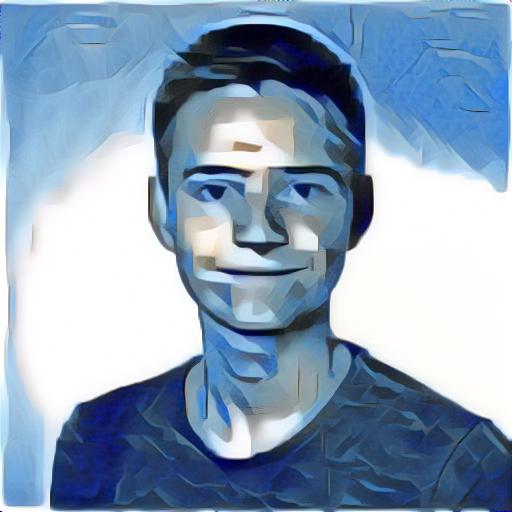
My research focuses on anomaly detection in high-dimensional data with deep learning models, and more recently with neuro-symbolic methods, mostly on image, video and text data. I also did some work on reproducibility of experiments involving deep learning models, as well as mining of academic literature.
I maintain pytorch-ood, a Python library for out-of-distribution detection with deep neural networks.
In my spare time, I like to scrape data from the web and mine it. This hobby spawned some interesting projects with dedicated websites, such as sworm and extra-mining.
On the right, you can find a rather dated picture of me in the style of an image from Picasso’s blue period. It was generated with Neural Style Transfer.